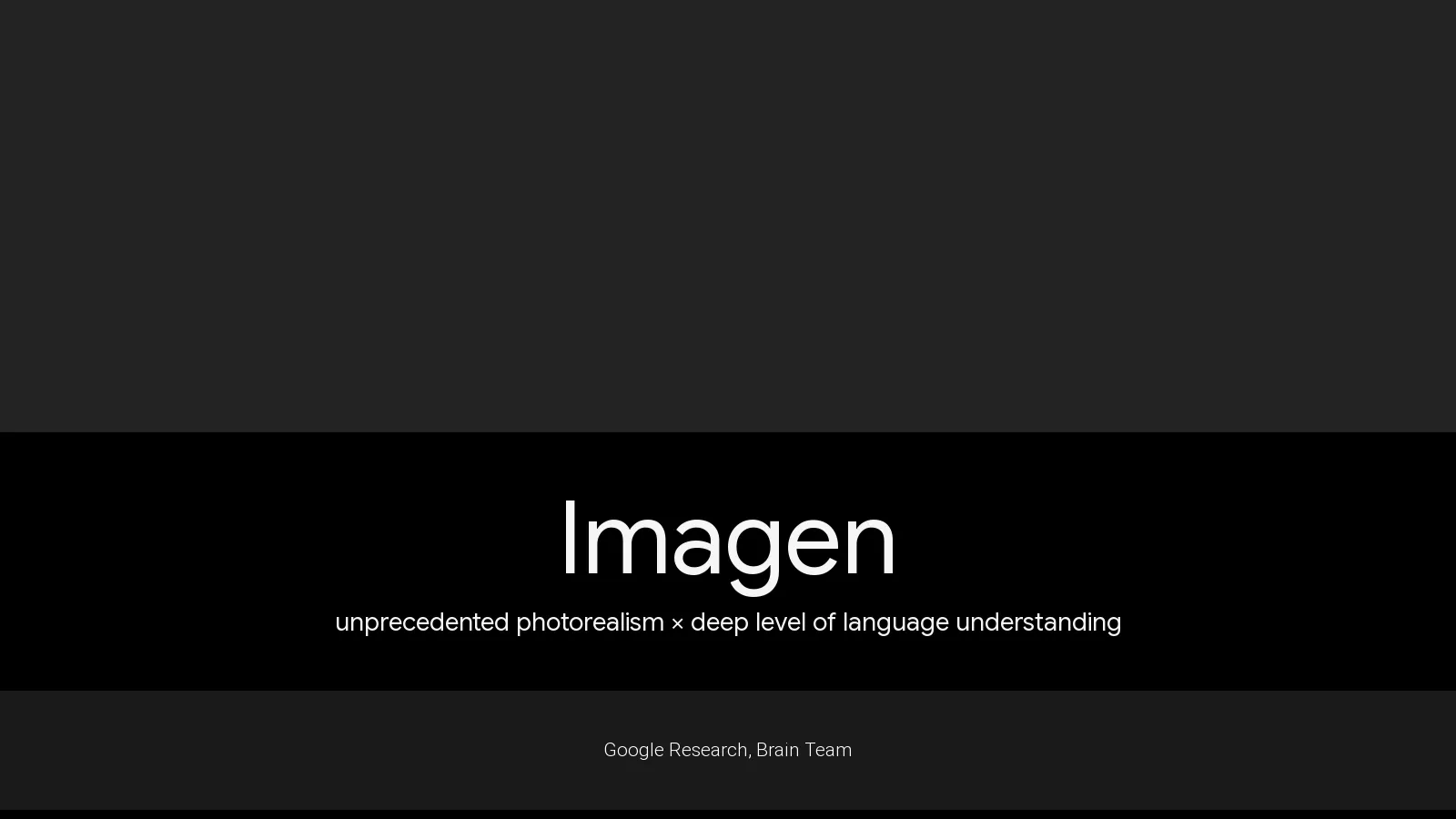
Imagen AI Details
Product Information
Category
Text-to-Image ConversionProduct Description
Imagen is a text-to-image AI system that generates photorealistic images from input text. It is trained on massive datasets and achieves state-of-the-art results in image fidelity and text-image alignment.
FAQFAQ
Website Traffic
No Data
Alternative Products
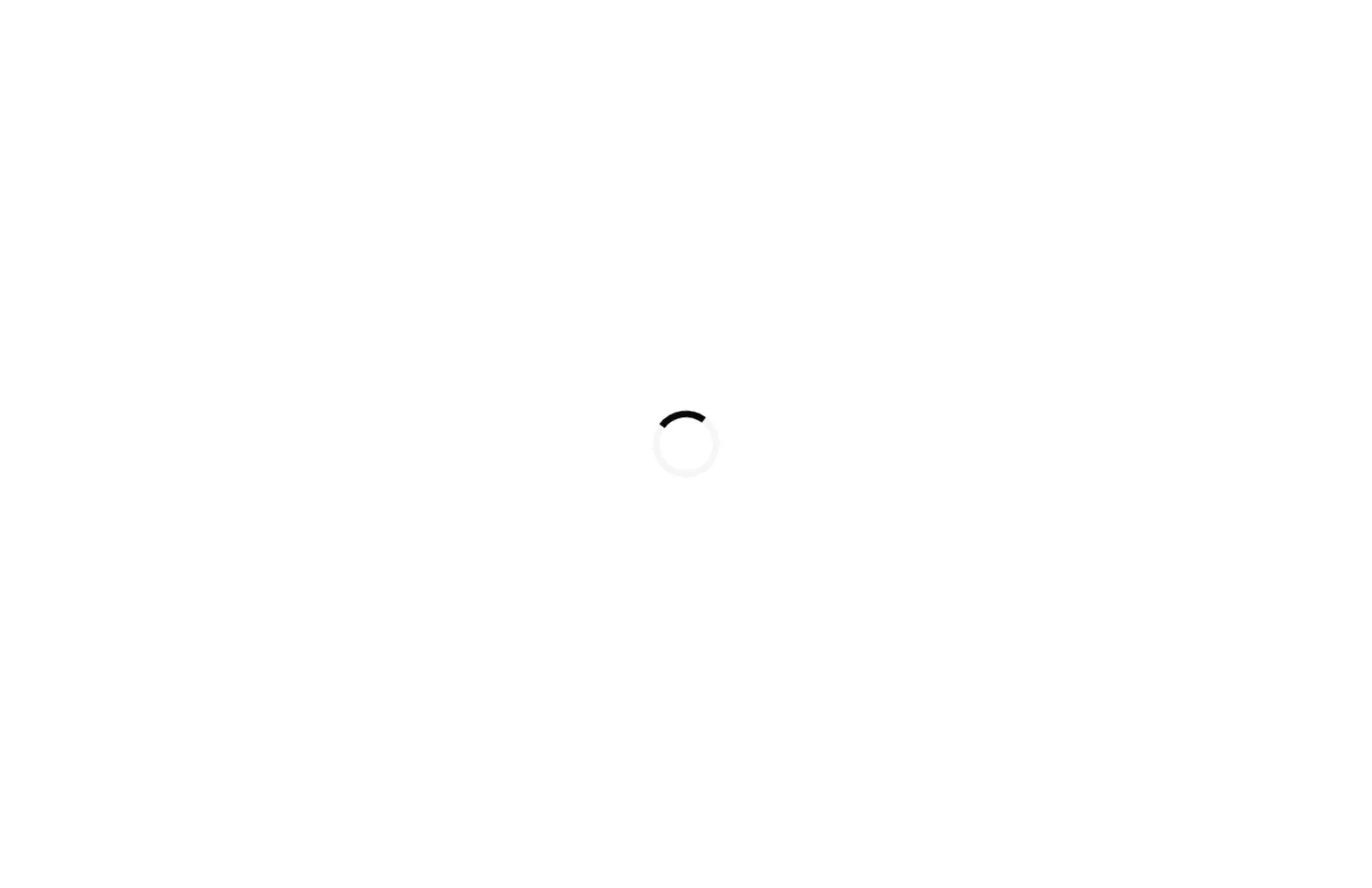
绘AI
Image Generation
Ai Drawing
1920
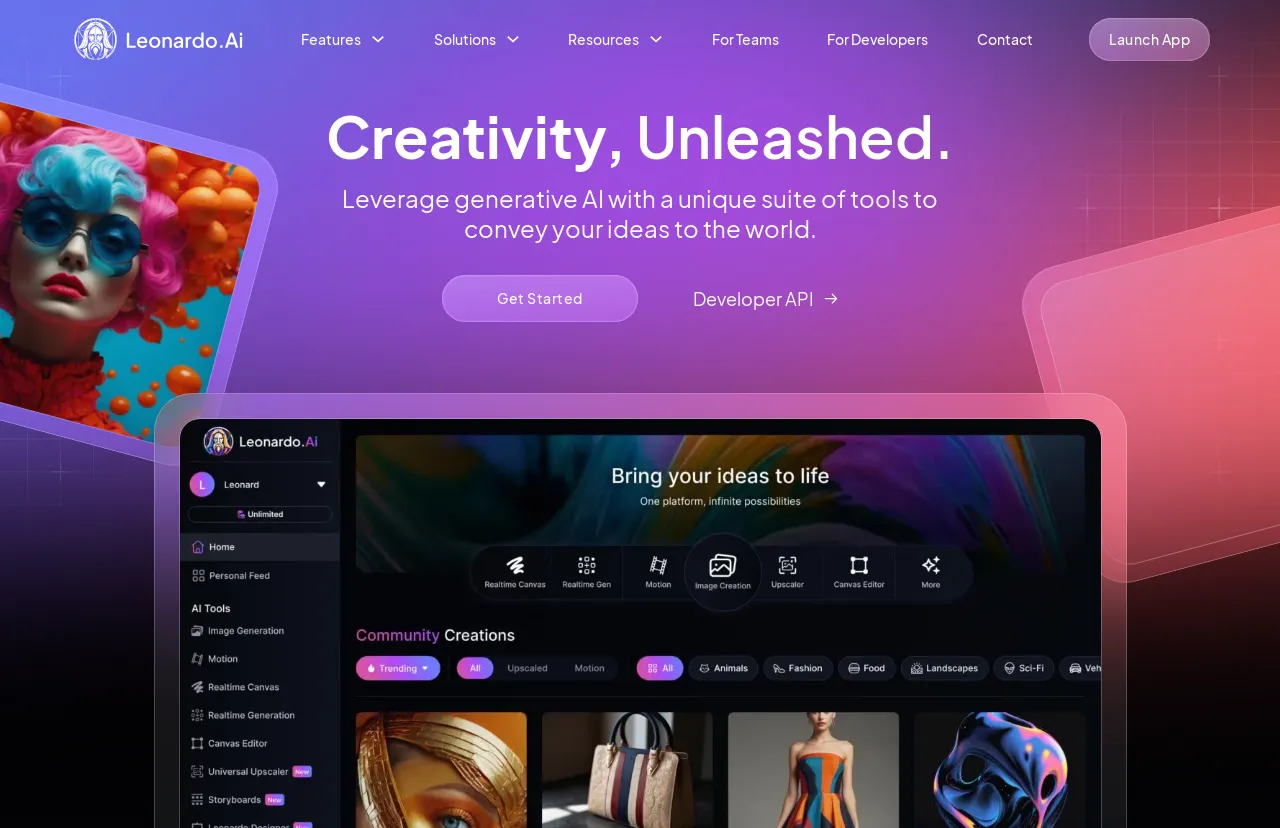
Leonardo AI
Image Generation
Creativity, Unleashed. Empowering your creative vision with generative AI.
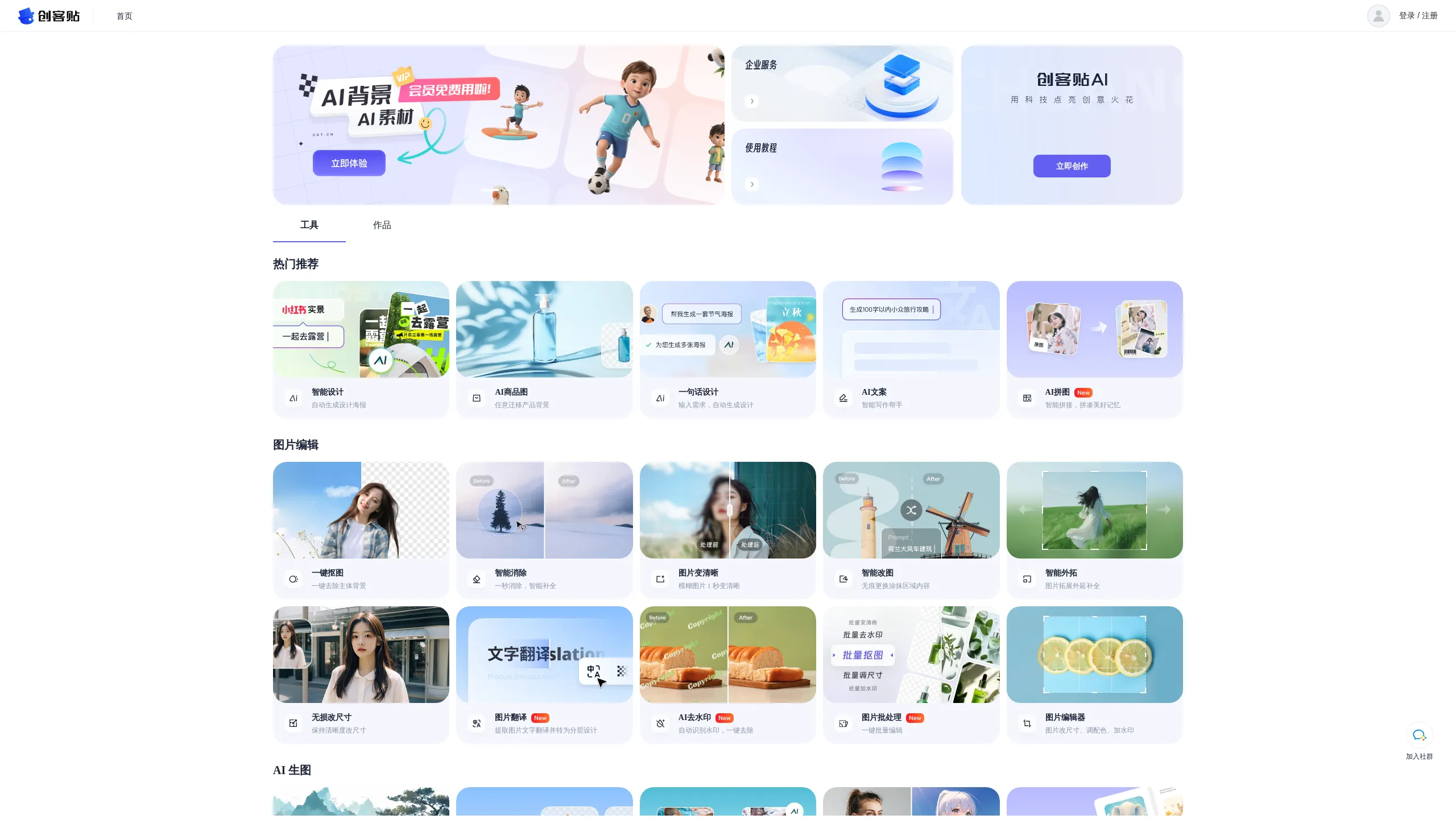
AI Art
Image Generation
AI Graphic Creation Platform
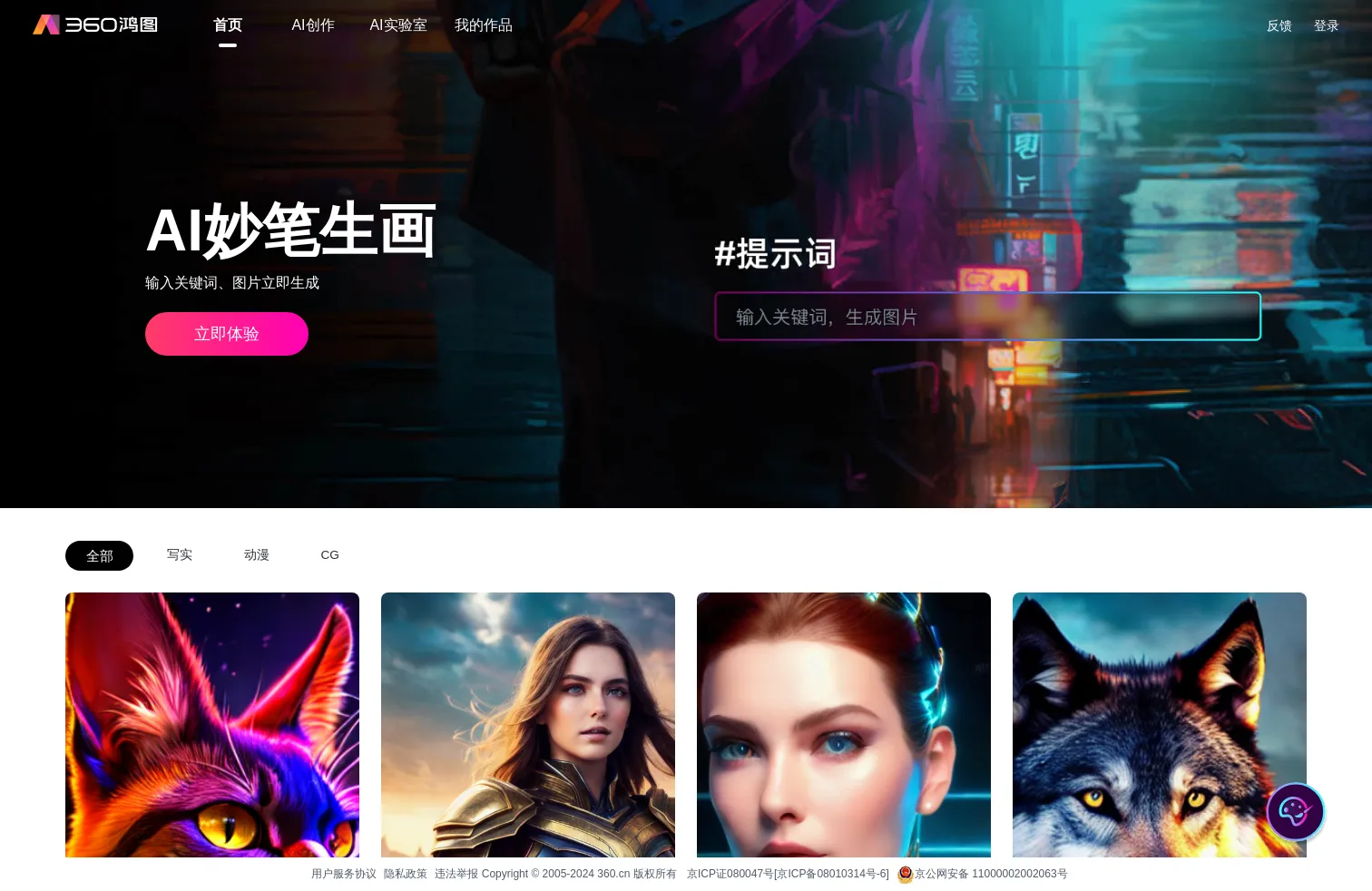
360 AI
Image Generation
AI Creates Stunning Artwork
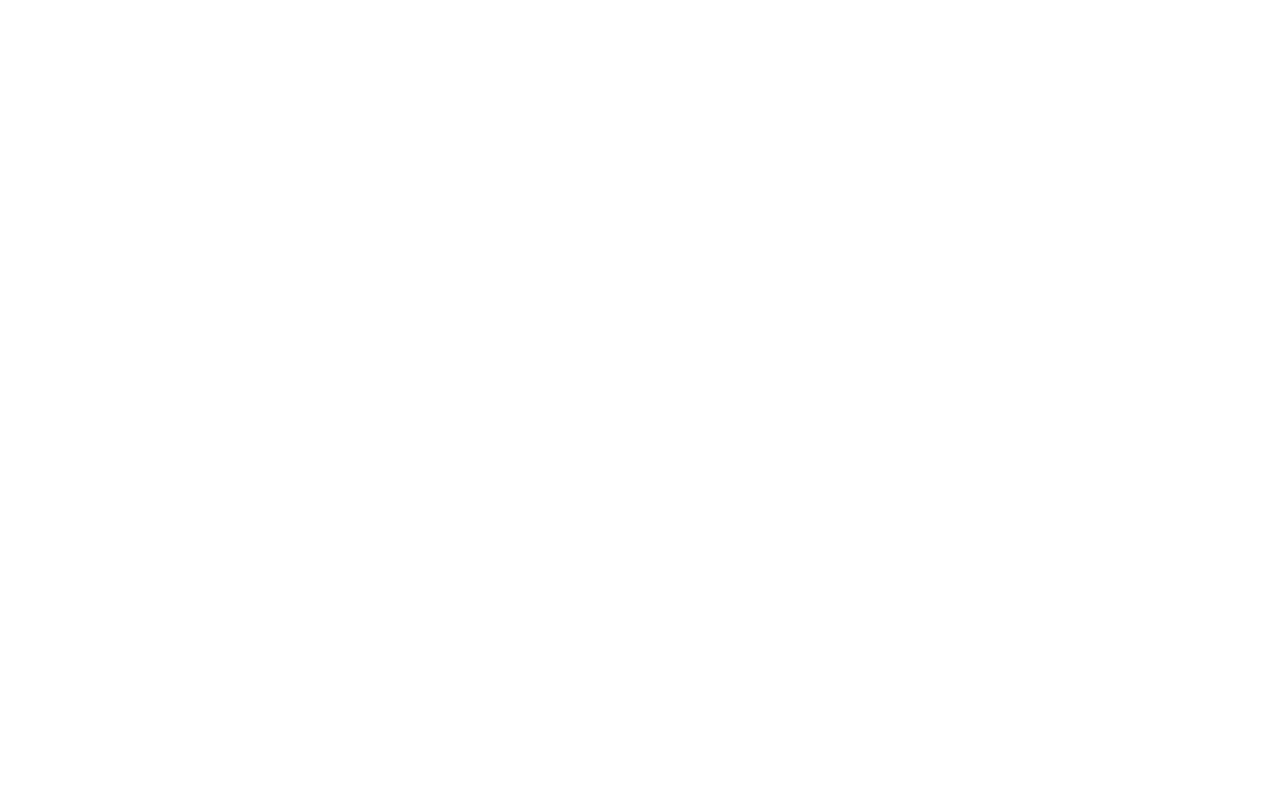
Stockimg AI
Image Generation
Stop wasting time on content production. Try it for free right now and manage your social media with AI!
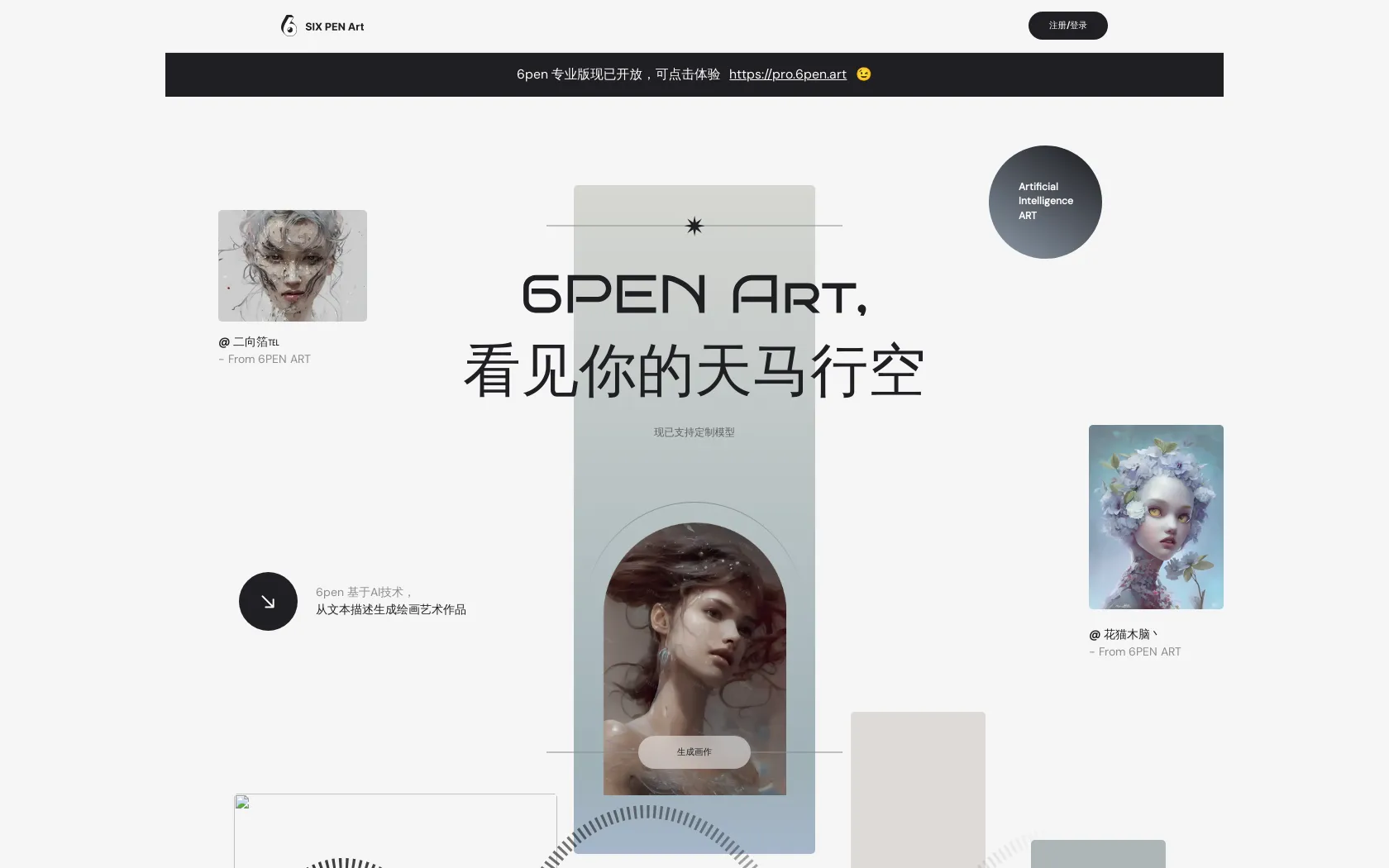
6pen Art
Image Generation
Turn your imagination into art